- A new non-peer-reviewed paper says that the equations of physics follow a formula of their own.
- The authors say their probabilistic idea can be used to help train physics machine learning.
- That said, their goals don’t match the actual use of AI.
In a real head-scratcher, scientists have applied a word frequency theory to three sets of physics equations and drawn several conclusions. When talking to Young scientist about the project, one researcher said he believes the work could inform machine learning algorithms aimed at finding new equations that model physics. Now the question must be asked: is this secretly the next Golden Report, or does the emperor have no clothes?
The researchers present their idea – which is detailed in a non-peer-reviewed study that appears now as a preprint in arXiv—explaining that it was inspired by something called Zipf’s Law. This law, originally conceived by linguist George Kingsley Zipf in 1935, states that word frequency functions like an inverse exponential distribution with a very long tail. The total incidence of a word is approximately equal to 1 divided by its rank in the text’s frequency graph. The most frequently used word is about twice as frequent as the second most frequently used word, and so on. Even though it has “law” in its name, that’s definitely not the best descriptor. Zipf’s law produces a measure of statistical “fit” and meaning, with definitions including “approximately” or “often”. It can certainly be useful in the right context, but it’s not an equation or a sure thing.
Physicists Andrei Constantin, Deaglan Bartlett, Harry Desmond and Pedro G. Ferreira decided to work out some physics equations for the frequency of different operators, and they report in their paper that these equations also obey Zipf’s law. This sounds exciting, but there are some really major caveats, one of which is that the group studied only three sets of equations. One was along the lines of a list of named equations from Wikipedia (which has, at most, about 250 equations not all related to physics). The researchers used 41 of these equations in their work. The other two sets they studied have 100 formulas (from the iconic physicist Richard Feynman’s lectures) and 71 algebra expressions.
If you’re wondering why they picked just 41 things out of a list of close to 150 or more related to physics, it’s because of another big caveat: the researchers eliminated a large number of expressions based on operators that used, limiting what they selected for their final analysis to a very small group. The team said that all of this was still statistically significant — a mathematical term that means the study has enough items to be meaningful and not biased by its smallness — because they found the same qualities in “21 corpora of random expressions algebraic with the same sizes and complexity distribution as the Feynman corpus” that they “randomly generated”. They don’t say how those randomly generated groups were created.
With all these caveats, the data the researchers studied was based on a special adjustment of Zipf’s law—one that uses an exponential term instead of an inverse relationship like the classic Zipf. They conclude that this probabilistic law (because, like Zipf, this is never a sure or guaranteed thing) can illuminate “all physical laws” as a so-called “meta-law of nature” and explain something about “nature mode”. operand.” This may set off alarm bells if you are wary of attributing unscientific intentionality to how physics shapes our world.
The whole thing would just seem a little odd – foreign ideas have gotten more play, for sure – if it weren’t for some really weird code. “Such a measure has the potential to improve symbolic regression algorithms by helping to filter out unphysical expressions of high complexity and in general to help us discover new laws of physics,” the paper concludes. In other words, these researchers think their probabilistic approach can narrow down the massive lists of possible new laws of physics generated by “artificial intelligence” technologies like large language models and machine learning.
While it is true that we are in a heyday of AI with massive lists of potential new molecules and other scientific innovations, a theory that points to simplicity rather than complexity does not necessarily seem appropriate. Promising drugs found using models like this are usually very complex and targeted. The discoverer of the YInMn Blue pigment, Mas Subramanian, said Folk Mechanics Earlier this year, AI models in his field produced lists of many impossible molecules for those who knew what they were looking at. These models have value, but likely not in simplicity, predictability, or even immediate practicality.
If there’s a nugget to sift through the billions of candidate pebbles, so far, there’s no clear best practice for narrowing them down using something like a probabilistic law. So far, the novelty that people appreciate in computing AI science is inherent in its non-humanity. These researchers have tried to eliminate it using an observed frequency theory, but looking for a pattern in this way is something the human mind already does. The advantage of AI is that it doesn’t need to be “narrowed down” using any of our little human notions.
Without its application to machine learning, what is the point of a principle that models “roughly” what the 200 or so general equations of physics say or involve? Of course something that is ‘often’ or even ‘sometimes’ true can be universally ‘sometimes true’, because that doesn’t mean anything in particular.
Even Zipf’s application in computational terms has adjustments, because you may have noticed something about how the law works: otherwise it would say that the most common word makes up 1/1 of the total, which is 100%. The law describes a relationship between words rather than their relationship to the overall text.
Anything that doesn’t fit Zipf can be grouped with other exceptions, and anything that Zipf confirms is kept as evidence. It is arguable that it verges on pseudoscience to use a linguistic idea like this as the basis for statistical analysis of a much smaller and narrower data set than physics. It looks like, unfortunately, our emperor may have been missing some important clothing after all.
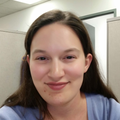
Caroline Delbert is a writer, avid reader, and contributing editor at Pop Mech. She is also an enthusiast for everything. Her favorite subjects include nuclear energy, cosmology, everyday mathematics, and the philosophy of it all.